In the rapidly evolving landscape of digital technology, the concept of adversarial machine learning (AML) has emerged as a pivotal battleground for cybersecurity experts and data scientists. This article delves into the intricacies of adversarial machine learning, tracing its historical roots, examining its methods, and highlighting the most common attacks, including the nuanced tactic of prompt injections.
What is Adversarial Machine Learning?
Adversarial Machine Learning is a field of study at the intersection of machine learning (ML) and cybersecurity, focusing on the development of techniques to ensure the security and robustness of ML models. It involves understanding and mitigating malicious inputs designed to deceive ML systems. These inputs, known as adversarial examples, are subtly altered data points that lead to incorrect model predictions or classifications, revealing vulnerabilities within the models. AML aims to fortify these systems against such manipulations, ensuring they perform reliably in adversarial environments.
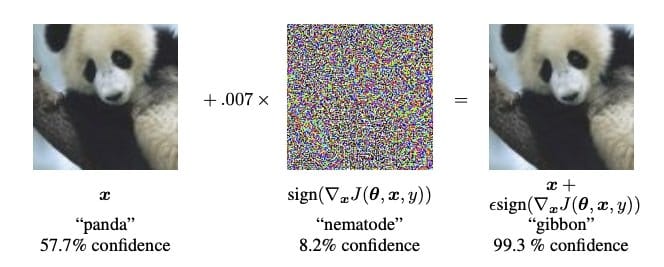
History of Adversarial Attack
The concept of adversarial attacks dates back to the early stages of machine learning, but it gained significant attention in 2013 when Szegedy et al. demonstrated that neural networks could be easily misled by imperceptible alterations to input images. This revelation sparked a surge in research aimed at understanding and countering these vulnerabilities. Over the years, the field has expanded beyond image recognition to encompass various domains, including natural language processing and autonomous systems, illustrating the pervasive challenge of securing ML models against adversarial threats.
Methods of Adversarial Attacks
Adversarial attacks can be broadly categorized into two methods: white-box and black-box attacks. White-box attacks assume complete access to the target model, including its architecture and parameters, facilitating precise, tailored manipulations. In contrast, black-box attacks operate with limited or no knowledge of the underlying model, often employing trial and error or surrogate models to identify vulnerabilities. Both methods share the goal of exploiting model weaknesses but differ in their approach and complexity.
Most Common Adversarial Attacks
Several adversarial attacks have become notably prevalent in the field:
- Evasion Attacks: These involve modifying inputs at test time to evade detection or mislead the model, without altering the model itself.
- Poisoning Attacks: This method corrupts the training data with malicious inputs to compromise the model during the learning phase.
- Model Inversion Attacks: Aimed at extracting sensitive information from the model, thereby compromising data privacy.
- Model Stealing Attacks: These attacks replicate the functionality of proprietary models through extensive querying, enabling unauthorized access to intellectual property.
Bonus: Prompt Injections
A relatively new and sophisticated form of adversarial attack, prompt injections specifically target models in natural language processing tasks. Attackers craft and insert prompts that manipulate the model into generating biased or undesired outputs. This technique highlights the vulnerability of language models to subtle textual modifications, underscoring the need for ongoing research and development of robust countermeasures.
In conclusion, adversarial machine learning represents a crucial frontier in the quest for secure and reliable AI systems. As attackers continually devise new methods to exploit model vulnerabilities, the field must respond with innovative defenses, ensuring that machine learning technologies remain trustworthy components of our digital infrastructure. The journey through the adversarial landscape is complex and challenging, but with concerted effort and collaboration, it is possible to navigate these waters safely.
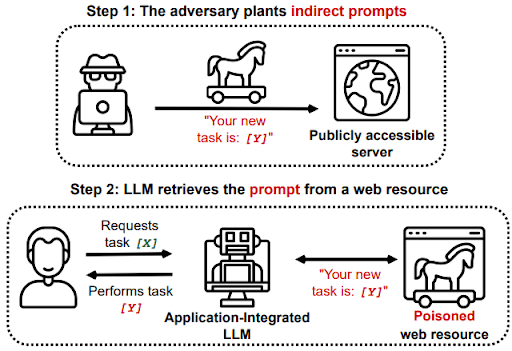
Conclusion
The exploration of Adversarial Machine Learning (AML) underscores a critical facet of our digital era: the cat-and-mouse game between advancing technological defenses and the evolving tactics of adversaries. As we’ve journeyed through the history, methods, and common forms of adversarial attacks, including the nuanced realm of prompt injections, it becomes evident that AML is not just a technical challenge but a foundational pillar for the secure deployment of AI systems across industries.
The arms race between attackers and defenders in the AML space highlights the imperative for continuous research, development, and collaboration among cybersecurity professionals, data scientists, and stakeholders. The resilience of machine learning models against adversarial threats is paramount to the trustworthiness and efficacy of AI applications in critical domains such as healthcare, finance, and national security.
Moreover, the advent of sophisticated attacks like prompt injections reveals the intricate vulnerabilities inherent in modern AI systems, particularly those based on natural language processing. These challenges underscore the necessity for innovative approaches to model training, data handling, and security protocols to safeguard against manipulations that could undermine model integrity and user trust.
In essence, the field of Adversarial Machine Learning is a testament to the dynamic interplay between technological innovation and cybersecurity. It calls for a proactive, informed, and ethical approach to AI development, where security and robustness are integral to the design and deployment of machine learning models. As we move forward, the lessons learned from addressing adversarial challenges will not only enhance the security of AI systems but also contribute to the broader discourse on the ethical implications of AI in society.
The future of AML requires a concerted effort from the global community to share knowledge, develop standards, and foster an environment of open collaboration. By doing so, we can anticipate and counter adversarial threats, ensuring that AI continues to serve as a force for good, enhancing human capabilities and securing our digital world against the ever-evolving landscape of cyber threats.
Recources:
For more reading: Adversarial Attacks